Current Projects
TwinSpace
The TwinSpace project aims to optimize (safety-relevant) software for embedded systems in terms of resource requirements (e.g. CPU, GPU, runtime). For this purpose, an automated reverse engineering of compiled code is intended. This code is then simulated on various emulated hardware (HW) platforms and the resource requirements are measured. As soon as the appropriate HW platform is selected, the code is recompiled for the corresponding HW platform. Traditionally, resource optimization for software (SW) is ignored, as the speed of HW doubles every two years anyway. By means of the developed, tested and applied green coding development tool with optimized resource management - TwinSpace - the SW development in the (safety-relevant) mass business of embedded systems is to be revolutionized. This will optimize both economic aspects, for example through more efficient development processes, and ecological aspects through increased energy efficiency or CO2 savings through smaller chip areas. The contributions of ISSE are outlined in three aspects:
- Process modelling for the new processes created by TwinSpace.
- Management concept for process execution and artifacts in TwinSpace.
- Evaluation concept for process artifacts in TwinSpace.
Contact: Dr. Christoph Knieke
DGT: Digitized Green Tech
6RLogistics
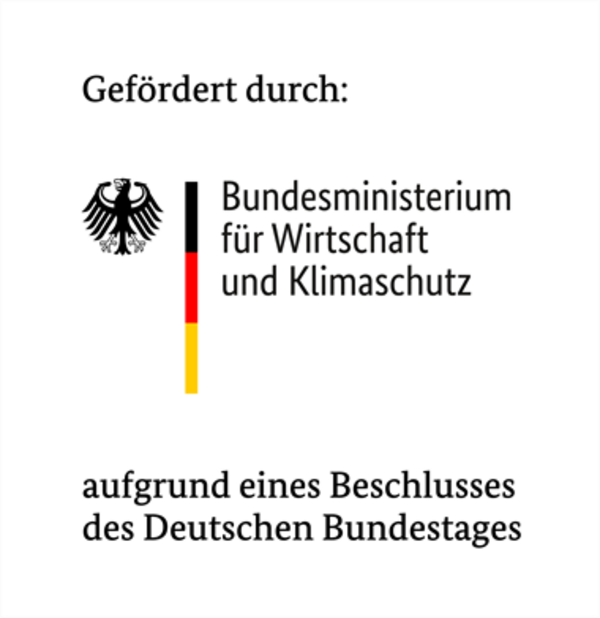
Deutschland hat sich mit dem 2021 beschlossenen Klimaschutzgesetz zu einer Verschärfung der Klimaschutzvorgaben verpflichtet.[1] Die Förderung der Kreislaufwirtschaft stellt hierzu einen grundlegenden Baustein dar und ermöglicht die aktive Abfallvermeidung und Wiederverwendung sowie die Rückgewinnung von Materialien durch Recycling.[2] Großes Potenzial bieten in diesem Sinne Elektro- und Elektronikaltgeräten, EAG genannt, da hier mit einer wachsenden Menge in den nächsten Jahren gerechnet wird und die Produkte sich aufgrund ihres Aufbaus oftmals für die Wiederverwendung und Reparatur eignen.[3] Allein 2018 wurden in Deutschland pro Person 10,3 kg entsorgt, womit Deutschland über dem EU Durchschnitt liegt.[4] Dabei werden immer noch EAGs unsachgemäß im Haushaltsmüll entsorgt, was wiederum die sachgerechte Wiederverwertung und das Recycling unmöglich macht.[5] Das Projektvorhaben 6RLogistics greift diese Problematik auf und schafft mittels künstlicher Intelligenz und smarter Logistik Abhilfe bei der Verwertungsentscheidung und dem Transport bzw. der Entsorgung. Dabei erfasst die künstliche Intelligenz den Zustand des Produktes bei den Nutzern und wählte darauf basierend den geeigneten Verwendungszweck. Daran angeschlossen erfolgt der Transport des Produktes vom Nutzer zu den Verwertern. Es findet daher ein Pull-Effekt durch die Verwerter statt, bei der die Idee des „Abstellraums“ als dezentrales Waren- und Ersatzteillager genutzt wird. Hierdurch wird nicht nur die Sammelquote in Bezug auf EAGs erhöht, sondern auch die Wiederverwendungs- und Recyclingquoten der Geräte, womit ein aktiver Beitrag zu den Klimazielen durch die Reduktion von CO2-Emissionen, sowie die Energie- und Ressourceneinsparungen geleistet wird. In drei verschiedenen Use-Cases werden die Aspekte eines solchen Systems im Rahmen des Vorhabens auf verschiedenen Ebenen erprobt und die Teilsysteme getestet. Dabei werden sowohl die Rückführung bei Endnutzern und in Annahmestellen untersucht, als auch die Erkennung und Evaluierung im industriellen Kontext. Somit werden im Rahmen des Vorhabens neue Systematiken für die Warenlogistik und Wiedernutzung von Gütern erprobt, welche dadurch der Kreislaufwirtschaft einen Vortrieb leisten.
PROJEKTPARTNER: eds-r GmbH (Nürnberg), ceconsoft GmbH (Goslar), Arbeitskreis Recycling e.V. (Herford) und Meikowe Elektro- und Teleservice GmbH (Fleringen).
Ansprechpartner:
E-Mail: Dominique Briechle
[1]www.bundesregierung.de/breg-de/schwerpunkte/klimaschutz/klimaschutzgesetz-2021-1913672
[2] Abfallvermeidung, Umwelt-Bundesamt, www.umweltbundesamt.de/themen/abfall-ressourcen/abfallwirtschaft/abfallvermeidung
[3]www.europarl.europa.eu/topics/de/article/20201208STO93325/elektroschrott-in-der-eu-zahlen-und-fakten-infografik
[4]www.destatis.de/DE/Presse/Pressemitteilungen/Zahl-der-Woche/2021/PD21_11_p002.html
[5]deutsche-recycling.de/blog/e-schrott-elektroaltgeraete-recycling/
Life_Twin
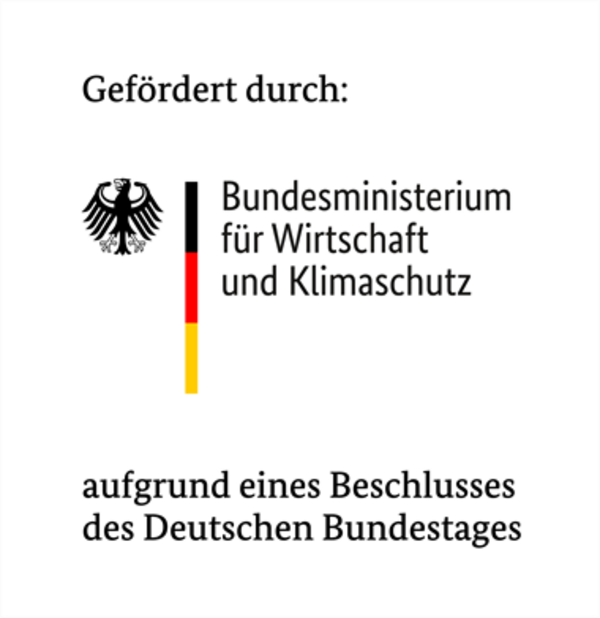
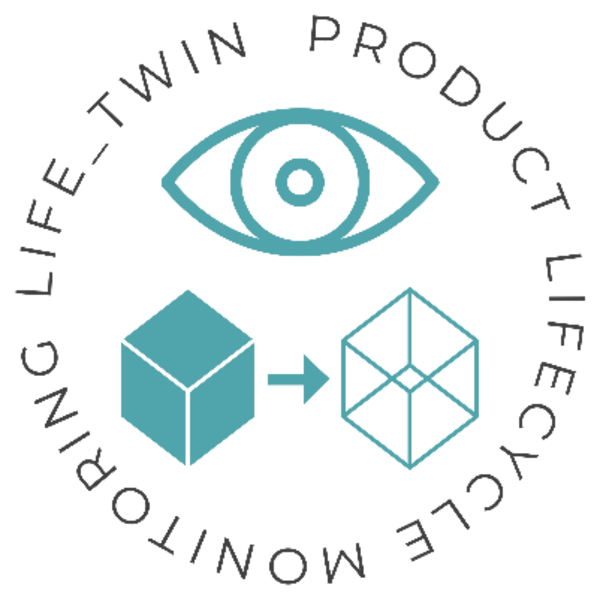
The electronic products currently in use are designed for only short-lived use cycles and are contributors to the current increase in global waste volumes and excessive use of raw materials. [1] As a result, electrical and electronic products generate emissions that can be reduced through repair and reuse. However, emissions are also generated during the storage, sale and disposal of equipment. Particularly in view of global warming, these emissions represent a major potential for reduction. The aim of the project Life_TWIN is to investigate smart solutions for condition assessment and to identify opportunities for reuse, thereby reducingCO2 emissions. The project proposal within the funding line "Resource Efficiency in the Context of the Energy Transition" within the 7th non-nuclear funding program of the German Federal Ministry of Economics and Climate Protection (BMWK) is thus in direct line with other research projects to pave the way for the Circular Economy. Research into solutions for improving the condition monitoring of products plays a central role in this, as it enables damage and material fatigue to products to be detected. Digital twins are to be used in the project, which will enable the condition of products to be recorded and controlled.
PROJECT TPARTNERS: IMW TU Clausthal, Bernhard Olbrich Elektroinstallationen-Industrieanlagen GmbH, Robert Bosch GmbH, Hellmann Process Management GmbH & Co. KG
Contact:
E-Mail: Dominique Briechle
[1] V. Forti, C. P. Balde, R. Kuehr, and G. Bel, "The Global E-waste Monitor 2020: Quantities, flows and the circular economy potential," 2020.
HitchHikeBox
In addition to environmental and health damage caused by emissions such as noise, CO2, and air pollutants, the quality of life is also increasingly restricted by road traffic due to a large amount of land used (e.g., for roads, parking areas, loading/unloading areas). With the progressive growth in transportation, especially in commercial logistics transport, the situation has become even more acute in recent years. The development of technical, urban planning, and social concepts to satisfy society's mobility and logistical needs while also increasing environmental protection and urban quality of life is THE challenge of the next decade.
In the project HitchhikeBox, an intermodal hitchhike logistics system based on AI-based journey planning of self-organizing delivery boxes is to be developed. This is a technological platform for an integrated electric mobility and logistics system that enables reduced private/commercial trips while maintaining mobility and logistics, as well as reduced parking space requirements, by maximizing the efficiency of current vehicles without sacrificing flexibility. The main innovation of the project is bringing forth an automated, decentralized dispatching system for self-organized "intelligent" logistics boxes (HitchhikeBox) that enable intermodal Multi-Hop-Routing “hitchhiking” via local Micro-Hub-Depots. Moreover, it aims to increase the use of all-electric vehicles by participating regional vehicle operators and to take advantage of synergy benefits by bundling individual journeys that can be coordinated. The Institute for Systems and Software Engineering (ISSE) is developing a software solution for the “intelligent” reusable logistics box for its independent and automatic hitchhiking from an initial to the final point. This solution will have intelligent strategies for the execution of a transportation task. Also, the development and conceptual design of this dynamic-adaptive self-organizing system based on smart contracts.
PROJECT PARTNER: MoD Holding GmbH, TU Clausthal (Institute for Software and Systems Engineering), Institute for Enterprise Systems (InES) University of Mannheim, Brehmer GmbH & Co. KG, PrimingCloud GmbH, Blockchain Solutions GmbH, Overath GmbH
Contact:
E-Mail: Marit Briechle-Mathiszig
VEAL – Virtuelle Entwicklung und Evaluation der Akzeptanz von automatisierten Level-4-Fahrzeugkonzepten
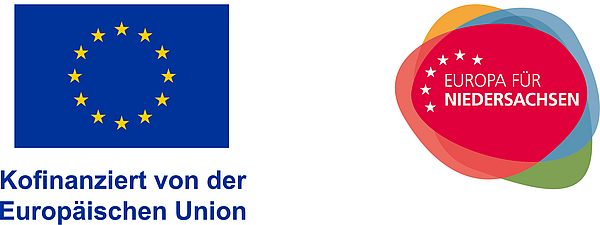
Das Verbundprojekt VEAL konzentriert sich auf die Entwicklung und Evaluation der Nutzerakzeptanz von automatisierten Level-4-Fahrzeugkonzepten, sowohl in virtuellen Simulationsumgebungen als auch auf realen Versuchsträgern. Das Institut für Software und Systems Engineering (ISSE) trägt die Verantwortung für das Teilprojekt der TU Clausthal, das sich auf die Entwicklung einer nutzer:innen-orientierten End-to-End-Learning Fahrfunktion für autonome, fehlertolerante (Fail-Operational) Systeme fokussiert. Diese Fahrfunktion wird in eine umfassende Fail-Operational Sicherheitsarchitektur integriert.
Um diese umfassenden Zielsetzungen im Teilprojekt zu erreichen, wurden mehrere Arbeitsziele definiert:
- Entwurf einer umfassenden Fail-Operational-Sicherheitsarchitektur.
- Gestaltung einer nutzer:innen-orientierten, KI-basierten End-to-End-Learning Fahrfunktion für autonome Systeme.
- Implementierung auf einer Entwicklungs- und Simulationsplattform.
- Etablierung eines Entwicklungsprozesses für die nutzerorientierte, KI-basierte End-to-End-Learning-Fahrfunktion als Blaupause.
Ansprechpartner:
E-Mail: Dr. Meng Zhang
GESAL: Ganzheitlicher Entwicklungsleitfaden für ein umsetzbares Sicherheits- und Automatisierungskonzept für Landmaschinen
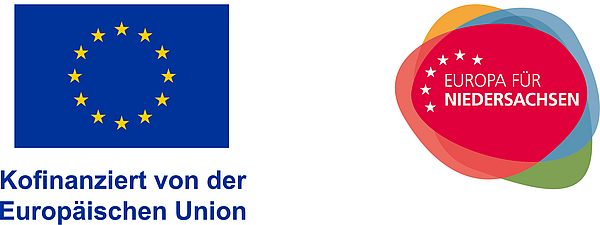
Das Hauptalleinstellungsmerkmal des Projekts GESAL ist die erstmalige Erstellung einer Entwicklungsblaupause, die ein ganzheitliches und umsetzbares Automatisierungskonzept mit expliziter Sicherheitsbetrachtung für Landmaschinen in den Bereichen Hardware-, Software- und Prozesssicherheit sowie Governance beinhaltet. Durch das Überwinden von Entwicklungshürden regionaler Unternehmen wird dadurch ein hohes Maß an Verwertbarkeit der Projektergebnisse sichergestellt.
Im Teilprojekt „C – Softwaresicherheit in automatisierten Landmaschinen“ des GESAL-Projekts wird das Institut für Software and Systems Engineering (ISSE) der TU Clausthal ein Absicherungskonzept für automatisierte Landmaschinen durch Softwarelösungen entwickeln. Dafür wird das ISSE eine Fail-Operational-Gesamtsystemarchitektur entwerfen, bestehend aus Softwarekomponenten samt ihren Schnittstellen, sowie eine Middleware-Lösung für automatisierte Landmaschinen. Zudem werden die definierten Softwarekomponenten auf eine im Teilprojekt A zu entwickelnde Hardwarearchitektur abgebildet. Hierbei wird die Umfeldsensorarchitektur aus dem Teilprojekt „A – Hardwaresicherheit in automatisierten Landmaschinen“ berücksichtigt. Die bestehenden Architekturansätze und die Expertise zur Realisierung der Fail-Operational-Maßnahmen für automatisierte Fahrfunktionen der Stufe SAE L4+ aus dem Automotive-Bereich werden in diesem Teilprojekt auf das landwirtschaftliche Anwendungsgebiet übertragen.
Ansprechpartner:
E-Mail: Dr. Meng Zhang
5GCampusKIShuttle
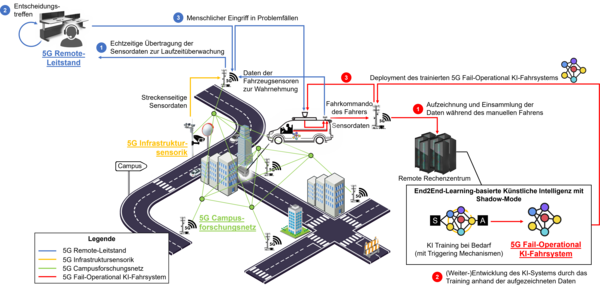
Der neue 5G-Kommunikationsstandard spielt eine entscheidende Rolle für innovative Anwendungen, insbesondere im Bereich des autonomen Fahrens. Dieses Projekt zielt darauf ab, ein 5G-Campusforschungsnetz zu etablieren, um die Anforderungen des autonomen Fahrens auf Level 3-5 ohne Sicherheitsfahrer an Bord und stattdessen mit einer technischen Remote-Aufsicht (Leitstand) zu erfüllen. Gemäß dem Gesetz zum autonomen Fahren der Bundesrepublik, das am 28.7.2021 in Kraft getreten ist, ist erstmals die Möglichkeit geschaffen worden, autonomes Fahren auf Level 3 ohne Sicherheitsfahrer und mit einer Remote-Aufsicht (im Sinne von einem 5G Remote-Leitstand im Projekt) im Regelbetrieb umzusetzen. Für diesen 5G Remote-Leitstand muss ein 5G-Netzwerk etabliert werden, das die erforderlichen Safety und Security-Eigenschaften des 5G-Funkstandards erweitert, um die Qualität des Funknetzes während der Teleoperation vorherzusagen und das Netz vor Angriffen zu schützen.
Zudem sollen autonome Fahrzeuge in der Lage sein, komplexe Fahrumgebungen zu bewältigen, wie etwa schlecht einsehbare Kreuzungen. 5G-Infrauktursensorik kann hierbei unterstützen, indem sie einen Ersatz für den analogen Verkehrsspiegel bietet, wie er von menschlichen Fahrern genutzt wird. Schließlich muss ein betriebssicheres KI-basiertes Fahrsystem (5G Fail-Operational) mit Shadow-Mode entwickelt und über 5G mit der 5G-Infrastruktursensorik und dem 5G Remote-Leitstand zu einem integrierten 5G-basierten Mobilitäts- und Logistikdienstleistungssystem verbunden werden. Ein autonomer CampusShuttle wird als Demonstrationsbetrieb aufgebaut. Das 5G-Campusforschungsnetz wird als Plattform und Testfeld für laufende und zukünftige Forschungsprojekte genutzt und steht den beteiligten Instituten und Zentren zur Verfügung. Die Projektergebnisse können als Modell für den Aufbau autonomer Shuttles in anderen 5G-Reallaboren und Gebieten mit 5G-Netzversorgung dienen. Das Projekt wurde in der vierten Beiratssitzung des Verbundvorhabens 5G-Reallabor in der Mobilitätsregion Braunschweig-Wolfsburg vorgestellt und einstimmig positiv unterstützt. Dies zeigt die ausgezeichnete Einbettung des Projekts in die 5G Innovationsregion Braunschweig-Wolfsburg.
Ansprechpartner:
E-Mail: Dr. Meng Zhang
Future Lab Mobility
The project of “Zukunftslabor Mobilität” (in English: Future Mobility Lab) focuses on the application-oriented research work by using different mobility-related technologies. In four well-defined interdisciplinary fields (Collaborative Research Fields (CRF 1-4)), potential environmentally and socially compatible mobility solutions such as connection of systems, human users and infrastructures relying on the digitalization technology will be investigated and developed, with consideration of concrete use cases about future mobility in Lower Saxony of Germany as well as related methods and approaches for the development of innovative mobility solutions.
In the scope of this project, TU Clausthal is involved together with other project partners in CRF 2, which focuses on relevant topics of smart mobility data handling. As known, the data plays a significantly important role as the basis of autonomous driving (CRF 1) and additionally as the precondition of the development and implementation of appropriate mobility services and corresponding business models (CRF 4). In CRF2, scientists from the research fields of communication technology, information and software engineering work closely together to develop methods to realize a safe and reliable data acquisition, evaluation and fusion of mobility data. For this purpose, the approaches for reliable (safety, security and privacy) data collection and processing, which fulfill the legal framework like DSGVO, will be developed and demonstrated with consideration of both phases for system development and operation. The approaches for the development of a reliable data architecture, methods and approaches for the standardization of data handling and fusion, as well as the assessment and assurance of data quality will be also developed and tested in the project.
Contact: e-mail: Abhishek Buragohain
InsectPassport
Project-Website: Link
The InsectPassport project aims to transform the insect industry by enhancing transparency in the food supply chain and developing a digital product passport to facilitate the acceptance of insect-derived protein from developing countries. This concept promotes sustainability and profitability in equal measure for the end customers. The digital passport for insect protein meal is an innovation that will ensure compliance with European regulations on insect-meal production, increase acceptance of insect meals from developing countries, and make them more affordable than conventional animal products. Additionally, embracing the digitalization of the insect supply chain can efficiently improve traceability, sustainability, scalability, and compliance monitoring while optimizing production processes, improving consumer transparency, and enabling data-driven decision-making. As regulatory frameworks continue to develop, the insect industry is emerging as a vital part of future sustainable food systems.
Contact:
Prof. Dr. Benjamin Leiding benjamin.leiding@tu-clausthal.de
BIOMetrix
Project-Website: Link
The goal of the BIOMetrix project is to develop a recommendation and evaluation system for biodiversity measurement actions based on scientifically tested AI biodiversity measurement methods (e.g., audio AI, AI image recognition) for customer-centered productization aimed at providing optimized actions for species protection and/or ecological risk management for companies as well as governmental institutions. Within the frame of the DATIpilot Innovation Sprint, a prototypical implementation of the concept for at least one, ideally several use-cases including a successful pilot project for biodiversity measurement in a defined test scenario, is being carried out. To this end, existing data sources and AI models will be supplemented with self-collected data from multi-sensor systems and further AI models will be developed. In terms of content, we will integrate future users (e.g., re-naturalization projects, national parks and nature reserves, urban wild areas, landscape protection areas, compensation area owners, the agricultural sector, etc.) who want/need to carry out biodiversity measurements and evaluations as part of species protection or risk management measures, into the development and pilot project. Moreover, the first steps towards the scaling and commercialization of the system are being taken with the aim of a subsequent spin-off
Contact:
Prof. Dr. Benjamin Leiding benjamin.leiding@tu-clausthal.de
The Limits to Growth – Sustainability and the Circular Economy (MOOC)
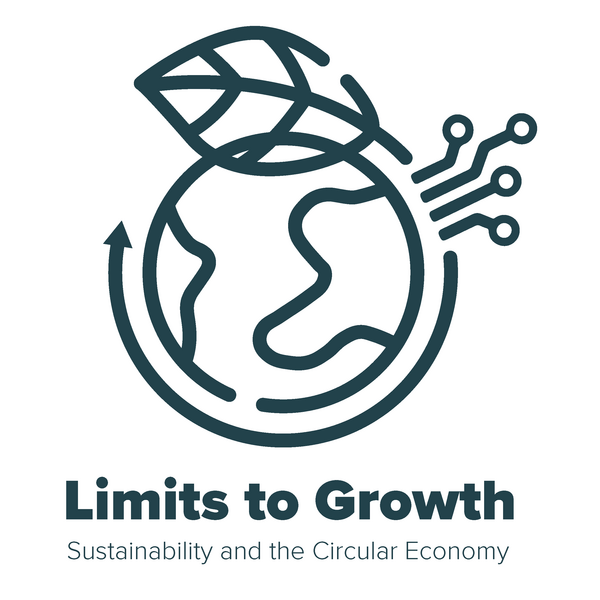
Project-Website: Link
In research, teaching, and technology transfer, the overarching theme for the Clausthal University of Technology, its guiding principle, is the Circular Economy. While degree-specific courses deal with selective aspects of the Circular Economy, there is a gap with respect to a course for all students that teaches the essential concepts and fundamentals of sustainability, environmental pollution, resource scarcity, and the Circular Economy.
The course “The Limits to Growth – Sustainability and the Circular Economy” aims to close this gap. As a general foundation course, there are no access restrictions. Furthermore, the course can be taken by Bachelor and Master students and PhD students. In order to accommodate a large number of international students, the course is conducted in English.
The Limits to Growth (LTG) course is open to all students of the Clausthal University of Technology as well as other organizations. Therefore, an adapted teaching format and appropriate technical support are required. The LTG course will be implemented as a Massive Open Online Course (MOOC), which scales traditional forms of knowledge transfer (slides, recordings, etc.) via an IT-driven infrastructure and expands them with additional technical features such as forums, quizzes and other forms of (semi-) automated tasks or tasks to be assessed via peer feedback. In addition, corresponding MOOC platforms offer participants interaction and networking opportunities and the possibility of asynchronous learning, in which students study according to their schedule. They are given access to all teaching and learning materials, which are discussed in regular meetings – followed by optional question-and-answer sessions to clarify any questions.
All teaching and learning materials, recordings, homework, tutorials, etc. are published under an open-source licence (CC-BY-SA-4.0) and are thus freely available as directly integrable teaching units or as a basis for further courses.
Contact:
Prof. Dr. Benjamin Leiding benjamin.leiding@tu-clausthal.de
SoRec – Digitalisation of Sorting Processes for Fine-Grained, Metal-Containing Waste Streams in the Recycling Industry
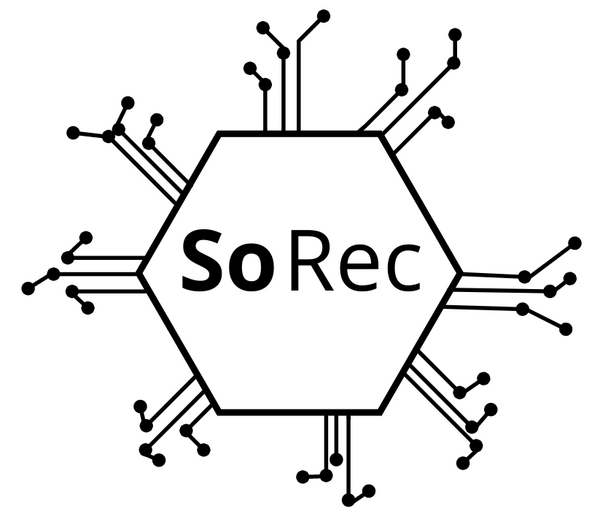
Project-Website: https://www.sorec-greentech.de/
Closing material and resource cycles is an integral part of the circular economy. Many products (especially electronic devices) are becoming increasingly complex regarding their structure and raw materials. To recover raw materials, the products must be broken down into ever-finier grain sizes and subsequently sorted. In general, dry sorting processes achieve good sorting results in the fine particle size range with lower throughputs. However, when increasing the throughput, the quality of the sorting results decreases considerably. In order to operate profitably, sorting machines must work as close as possible to the tipping point between good quality and maximum throughput while also minimising maintenance downtimes.
The SoRec project focuses on digitalising sorting processes for fine-grained, metal-containing waste streams in the recycling industry. By installing state-of-the-art industrial line cameras and sensors, we are digitizing the conventional sorting method on a moving belt. With the help of advanced AI models and algorithms in deep learning and machine learning, our system can accurately detect materials on the conveyor belt, classify them based on size, shape, and color, and even find their precise edges. With the capability to identify multiple layers of materials, the AI model provides valuable density and volume estimation. To ensure real-time efficiency and control, we have integrated the AI model with powerful computer vision techniques, which handle crucial image processing tasks. This seamless collaboration between AI and computer vision allows us to estimate the belt’s speed and detect any anomalies, ensuring precise sorting and preventing belt misalignments. Our materials, measuring just 1 mm in size, demand meticulous attention to detail, necessitating high-level zoom capabilities for precise annotations. With this innovative AI-driven system, we are taking a significant step towards automating and optimizing the sorting process, enhancing productivity, and elevating the industry to new heights of accuracy and efficiency.
Contact:
Prof. Dr. Benjamin Leiding benjamin.leiding@tu-clausthal.de
ML4E: Machine Learned Models for Engineers
Ernst-August-Grubenwassermonitoring (EAGruMo)
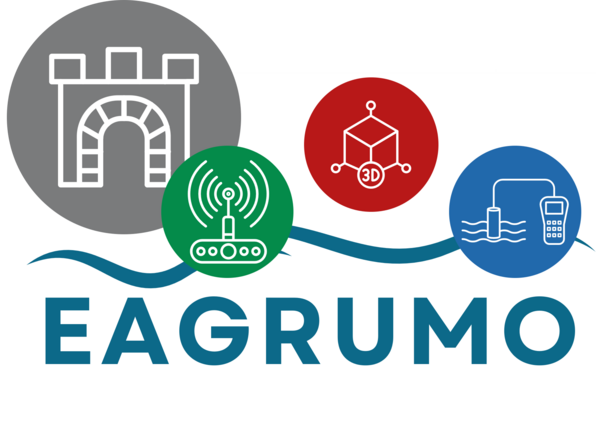
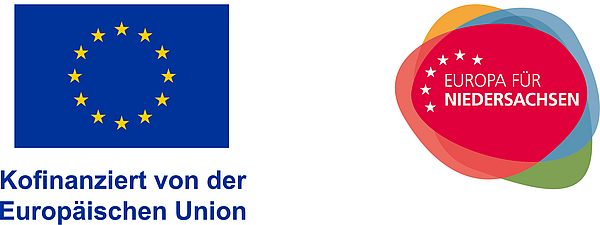
Das Projekt Ernst-August-Grubenwassermonitoring (EAGruMo) widmet sich dem langfristigen Erhalt der Wasserqualität und -verfügbarkeit im Harz, einer entscheidenden Region für Norddeutschlands Wasserversorgung. Aufgrund der Herausforderungen durch den Klimawandel und den steigenden Wasserbedarf der umliegenden Industrien wurde der innovative Einsatz des Ernst-August-Stollens als unterirdischer Wasserspeicher untersucht. Um das Potenzial dieses Stollens und anderer historischer Bergbaustrukturen zu ermitteln, ist das Projekt in drei Schwerpunkte unterteilt: die Entwicklung eines mobilen Multi-Sensor-Systems zur Erkundung von Gewässern, die Erstellung hydrologischer und hydrogeologischer Modelle und die Einführung eines kontinuierlichen Überwachungssystems für die Wasserqualität.
Im Rahmen des EAGruMo-Projekts ist das ISSE mit mehreren Schwerpunkten beteiligt. Im Arbeitspaket „Entwicklung eines mobilen Multi-Sensor-Systems (MSS) für die räumliche und qualitative Wassererkundung“ liegt der Hauptfokus des ISSE darauf, eine KI-gesteuerte autonome Navigation des MSS unter Verwendung des End-to-End-Learning-Ansatzes zu entwickeln. Diese Steuerungsmethode wird sowohl in virtuellen und realen Laborumgebungen als auch abschließend im Ernst-August-Stollen getestet. Innerhalb des Arbeitspaketes „KI-basierte Prädikation von Messwerten“ sucht das ISSE nach Möglichkeiten, Virtuelle Sensoren zu nutzen um mit Hilfe von Methoden der Künstlichen Intelligenz Messwerte zwischen den Befahrungen mit dem MSS zu erzeugen und um die Hydrologischen Simulationsmodelle aus den Teilprojekten der Anderen Partner für Echtzeitvorhersagen zu beschleunigen. Hierfür kommt der Ansatz der hybriden Modelle zum Einsatz. Dabei wird die KI nicht nur datenbasiert, sondern auch auf Basis von bekannten Gesetzmäßigkeiten der Ingenieure tariert und so auf physikalisch plausible Prognosen beschränkt.
Projektwebsite: https://www.eagrumo.tu-clausthal.de/
Ansprechpartner: Dr. Stefan Wittek
MOdellkopplung im Kontext eines VIrtuellen Untertagelabors und dessen Entwicklungsprozess (MOVIE)
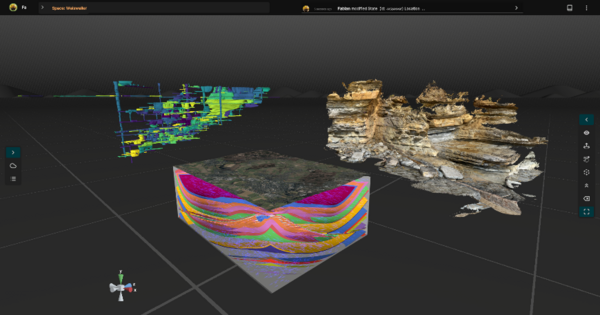
Im Bereich der untertägigen Bewirtschaftung haben bisherige Simulationsmodelle einzelne Aspekte wie Geologie, Hohlraumgeometrie, Wettertechnik/Strömungsmechanik und Geomechanik separat betrachtet. Eine umfassendere Modellkopplung ermöglicht jedoch eine ganzheitlichere Analyse der Systemzusammenhänge und der Abhängigkeiten der Modelle untereinander. Im Rahmen des MOVIE-Projekts wird ein hybrides Modell unter Einsatz künstlicher Intelligenz entwickelt, um die Rechenzeit für diese Simulationsmodelle zu optimieren und eine interaktive Nutzung im virtuellen Labor zu ermöglichen. Dabei wird ein retrospektiver Simulationsansatz verwendet, bei dem die Prognosen basierend auf historischen Daten mit aktuellen Messungen aus dem realen Untertagelabor verglichen werden. Die dadurch ermittelte Differenz wird zur Feinabstimmung des Modells genutzt.
Projektpartner: TU Clausthal - Institut für Bergbau (IBB), Institut für Endlagerforschung (IELF-LR und IELF-GM), Institute for Software and Systems Engineering (ISSE); Technische Universität Bergakademie Freiberg - Institut für Bergbau und Spezialtiefbau, Forschungs- und Lehrbergwerk (FLB) „Reiche Zeche“; fibrisTerre Systems GmbH (Berlin) und die Terranigma Solutions GmbH (Aachen).
Ansprechpartner: Dhananjeyan Jeyaraj
National Research Data Infrastructure for Engineering Sciences (NFDI4Ing)
NFDI4Ing brings together the engineering communities and fosters the management of engineering research data. The consortium represents engineers from all walks of the profession. It offers a unique method-oriented and user-centred approach in order to make engineering research data FAIR – findable, accessible, interoperable, and re-usable.The consortium represents researchers from all engineering disciplines. It offers a unique method-oriented and user-centered approach to make research data FAIR - discoverable, accessible, interoperable and re-usable.
NFDI4Ing defines a total of 7 archetypes for scientists as users of research infrastructure.The ISSE is mainly active in the BETTY archetype: engineering research software. This includes in particular the code of simulation models, and questions concerning the integration of heterogeneous models, as well as their approximation with the help of AI methods.
Contact: Vasiliy Seibert
KI4All
This project aims to develop subject-specific and interdisciplinary study and learning content on artificial intelligence, particularly in the field of machine learning, for all status groups in universities and to enable its use by external stakeholders (i.e. the interested public) across universities.
universities and to enable their use by external stakeholders (i.e. interested members of the public). As a decentralized platform for these coordinated development activities, an AI hub will be established, which will enable coordinated development and usage planning as well as the implementation of didactically secured and innovative learning and mediation concepts of methods and application-oriented tools in the context of data-driven modeling, analysis and simulation for different target groups and promote AI-based innovations through participatory formats.
Contact: Stefan Wittek
Trink Extrem
The aim of the research project is to develop methods, precautionary concepts and transferable practical tools for extreme events for water supply companies and the water management administration. In this context, relevant sub-areas of water supply from water extraction and treatment to operation and water use are addressed, as are the different types of raw water: groundwater, spring water and surface water. In representative model regions of the German long-distance and surface water supply, an exemplary implementation takes place. This allows further long-distance and surface water suppliers or local water suppliers to adapt their respective different local requirements in a practical way. Ultimately, the project should also provide impulses for rule-making, e.g. in risk management and for cooperation with state authorities in extreme situations. The project should also raise awareness for the challenges of water supply during extreme events. A modern public relations work shall sensitize water supply companies with comparable challenges as well as interested end customers for the topic of extreme events and show the possible solutions developed in the project.
Contact person: Sakshi Singh
CircularLIB
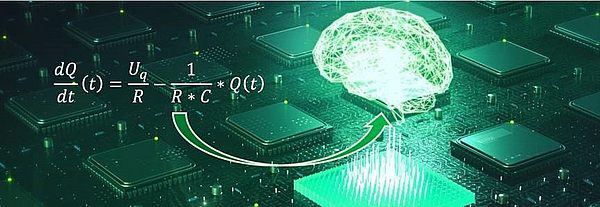
This project is part of the CircularLIB research training group on the cycle-oriented production of lithium-ion batteries. In this program the three universities TU Braunschweig, TU Clausthal and Leibnitz Universität Hannover are cooperating with the Fraunhofer Institute for Surface Engineering and Thin Films IST.
The aim is to research a hybrid AI-based modeling approach for lithium-ion batteries. Recently, machine learning has also been increasingly used in the context of classical engineering disciplines. In particular, deep learning approaches provide fast and relatively accurate modeling approaches based on learning specific tasks from examples. This is of crucial interest especially in the context of high-dimensional complex systems, where the underlying physics is not fully known or the computational effort for simulation with conventional numerical methods is high. Another aspect driving the use of deep learning approaches is the need for fast models that can be used in iterative tasks such as optimization and control. However, data-driven models are typically black-box approaches that are developed based only on data and do not explicitly incorporate physical knowledge or constraints into model development. This can result in such models lacking robustness and accuracy, especially with limited training data.
Recent advances in physically-informed machine learning have led to a number of approaches that are well suited for solving various types of partial differential equations (PDEs). This project aims to develop models based on both data and available physical knowledge for lithium-ion batteries using physically informed machine learning techniques that allow for a hybrid AI-based modeling approach.
Contact: Hamidreza Eivazi Kourabbaslou