ML4E: Machine Learned Models for Engineers
Machine Learned Models for Engineers
For complex technical systems, it is difficult to create complete physical models. Even if this succeeds, the computational power required for these models is often problematic. An alternative are models based on machine learning methods. In recent years, these have achieved astonishing success in predicting the behavior of such complex systems.
Even if no complete model of the system exists, engineers and scientists often have a great understanding of the physical and technical conditions underlying the systems.
However, this knowledge is not exploited by the black-box approach underlying classical ML models.
The goal of our group is to find hybrid ML approaches that exploit this knowledge to improve the quality of the predictions of these models.
In particular, we consider the question:
- How can existing physical (sub)models be integrated?
- How can domain knowledge be taken into account when training ML models?
- How can robust predictions be made despite sparse data?
- How can hybrid models strengthen the confidence in the predictions?
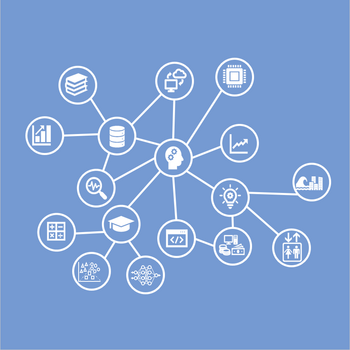